Using data to predict future risk of elderly patients in nursing homes
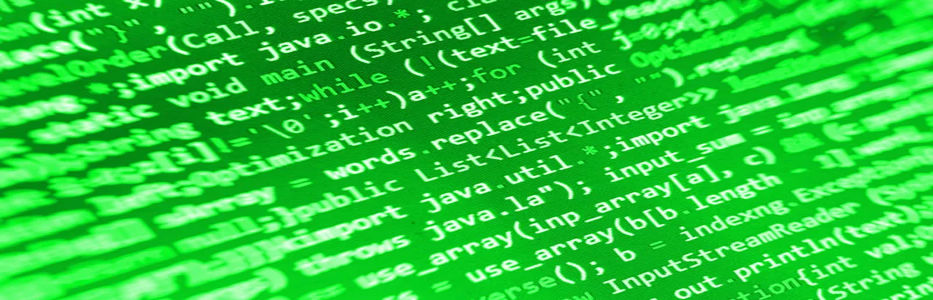
LONDON: New research shows how data can be used to predict future risk to elderly patients in nursing homes.
Robbie Felton grew up observing elderly care facilities and watching his mother, a social worker, on home care visits. She eventually rose to become executive director of her organization and now sits on the board of a national long-term care association. In his transition to college, and eventually studying public health at Brown University, Felton said he quickly realized there were limited data insights within elderly care facilities, and room for much improvement.
He and a team of three other Brown students (Alex Rothberg, Evan Jackson, and Samuel Prado) founded Intus Care, which uses data analytics to predict the future risk of elderly patients in nursing homes.
Jackson, the startup’s chief operations officer, told the Globe that Intus Care is already working with more than 20 facilities across Rhode Island, Massachusetts, Washington, and Michigan.
Q: What is Intus Care and how does it work?
Jackson: Intus Care is a health analytics platform that synthesizes data in long-term care facilities to empower providers to make data-driven management decisions for their elderly patients. We analyze the accessible health history of a patient in order to make predictions on future risk of adverse health events including hospitalizations, chronic disease onset, and more.
Q: What are some of the issues you are identifying?
Jackson: [Some of the issues include a] lack of interoperable software tools for long-term care providers to effectively leverage their data when providing care. There’s a lack of interoperability and standardization in health record data sources, which can create gaps throughout the health care system. The gaps in patient information can cause disruptions in the continuity of care, leading to a decrease in the efficiency of care delivery, miscommunications in care coordination and planning, over-utilization, and ultimately lower clinical outcomes.
Q: How does your system predict patient health and hospitalization risk?
Jackson: [Intus Care] predicts patient health risks by using machine learning to analyze the existing conditions of patients and how they impact the likelihood of an adverse health event. We examine a patient’s risk from a holistic perspective by incorporating factors that span from social determinants of health, active conditions, history of utilization, [among others].
Q: Predictive models already exist in the field. How is yours different?
Jackson: Our focus on predicting risk in elderly and complex patients sets us apart. Our model has been directly developed to analyze the comorbidities and clinical factors that impact [these patient groups].
Q: What challenges did you face in the development phase, and how did you address them?
Jackson: Finding our first customer. The key here was trust. Building trust with clients and people in the industry is important. And starting out, we had to get one organization to trust us enough to begin a working relationship so that they could inform us on how we could improve.
Q: What challenges will you face in the near future, and how will you address them?
Jackson: Scaling to meet the needs of a growing customer base. As we scale it requires increased attention to infrastructure including tech, data, and customer success. In order to meet these challenges, we have recently raised $1.65M to grow.
Q: What have you fundraised so far, and how?
Jackson: To date, we have raised $2.3 million across two fundraising rounds. We have raised capital through institutional investors including EO Ventures, Third Culture Capital, and Green Egg Ventures, through grants from winning the MassChallenge and Brown Venture Prize, as well through strategic angel investors including Brian McCarthy (Co-head of Healthcare Investment Banking at Bank of America) and Nancy Zimmerman (co-founder at Bracebridge Capital).
Q: What will your business model look like?
Jackson: We operate under a B2B subscription-based per patient model where we charge organizations on a per-patient per-month basis.